ARDentify platform for precise target identification
The Future of Immuno-peptidomics
ARDentify revolutionizes the field of immuno-peptidomics, enabling precise identification of peptides presented via HLA molecules on the cell surface. This high-accuracy solution delivers outcomes that significantly outperform standard methodologies.
ARDentify's technology is exceptionally apt for target identification, streamlining and enhancing both vaccine design and TCR-T cell therapies.
Experience the future of precision medicine, today with ARDentify.
Connect with us for a customized Demo
Identify the right therapeutic targets
presented via HLA-I & -II molecules
Enhance your experimental approach
with high accuracy in silico predictions
to select the right target epitopes
Whether you are developing gene and cell therapies or aiming to stimulate a patient’s immune system, you need to be sure that your therapeutics will have a chance to reach the selected target and will not affect healthy tissues.
Combine experimental methods with Ardigen's ARDentify platform - a computational approach to increase your chance of success.
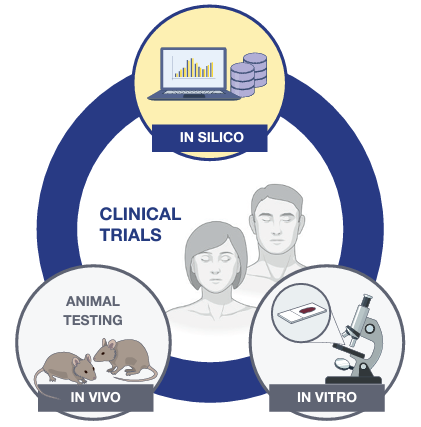
Did you know…?
As many as 70% of first-in-class drugs, which target an until-then unknown target or biological pathway, are identified through a target-based drug discovery strategy. At the same time, inappropriate target selection is often indicated as a major cause of experimental drug failures.
Before you invest additional resources in a target, it is important to provide as much evidence as possible in support of your choice, which can be approached by experimental methods or computational inference. Often a combination of those approaches is required to fully identify and understand the mechanisms of on-target and off-target effects.
Work with us to overcome the following challenges
Identify the right intracellular protein targets
by modeling the HLA-I presentation pathway
Identify peptide sequences
that have the potential to trigger
an immune system response
Almost every self-protein is represented on the cell surface. Endogenous peptides coming from proteolytic processing & degradation can escape further lysosomal processing. However, only a tiny fraction of protein fragments bind to HLA molecules, and an even smaller subset is presented on the cell surface and can be reached by T-cells.
Enable the detection of such peptides by using Ardigen's ARDisplay-I model - our methodology based on an in-depth understanding of the antigen processing and presentation pathways.
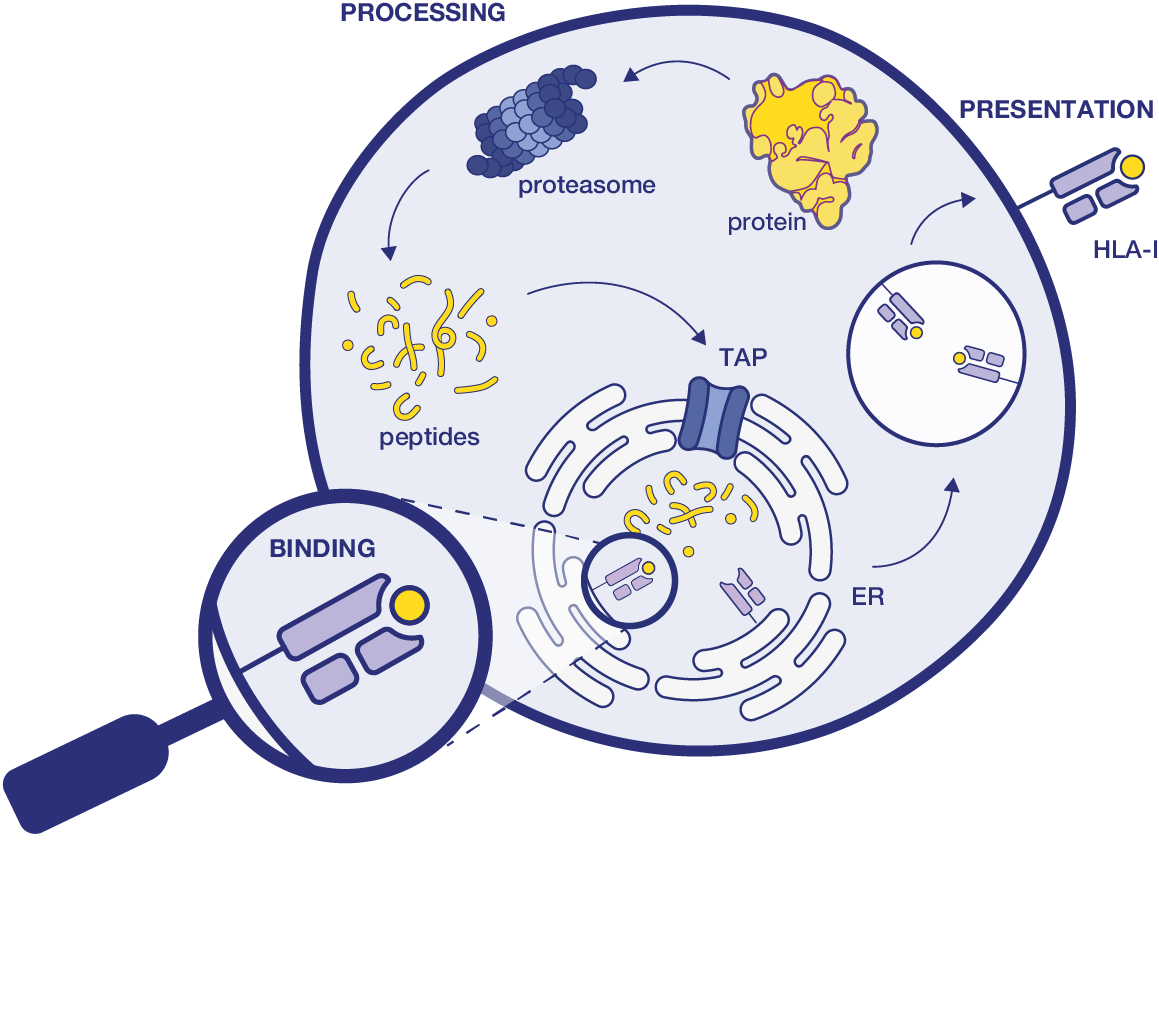
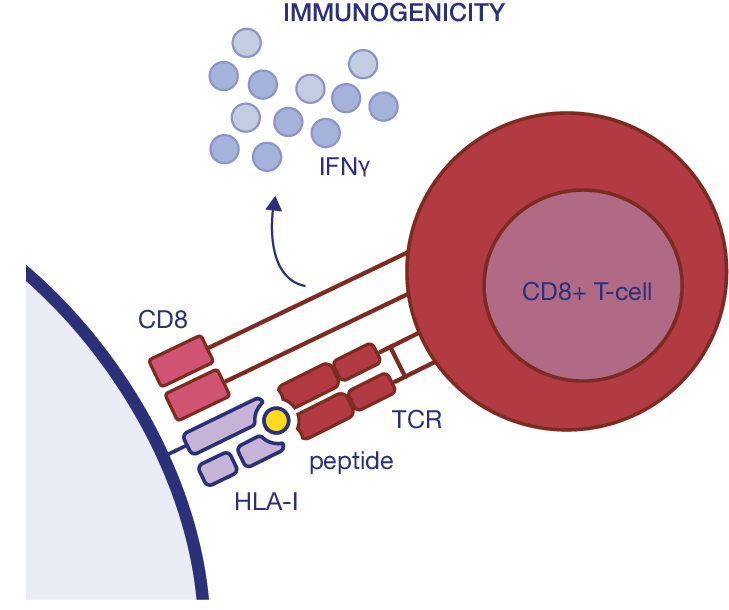
Check how we stand out from other solutions
Integrating data from various sources is essential to achieving accurate predictions and high-performance machine learning models.
● We combine non-presented artificially-generated samples, results from binding affinity (BA) assays, and information about mass spectrometry eluted ligands (MS EL).
● We incorporate MS EL from multiple high-quality sources (with over 2M unique peptides presented via 182 HLA alleles) and collaborate with an academic expert in immuno-peptidomics.
● Our data collection, comprised of single-allelic and multi-allelic samples, originates from EBV-transformed cell lines, cancer patients, and healthy donors.
Additionally, we provide a customized approach to data processing that includes filtering of positive observations, hard-examples mining, and biologically-aware generation of negative examples.
Ardigen's ARDisplay-I model enables prediction of HLA-I presented peptides with over 2 times higher Average Precision than standard solutions1
The study cohort2 includes 22 patients with CRC (colorectal cancer, adenocarcinomas) and represents a wide range of HLA alleles. It is comprised of 49 distinct alleles (HLA-A: 13, B: 20, C: 16), and almost 90% of the world population3 has at least 3 HLA alleles in common with these patients.
Comparison of precision-recall (PR) curves. Our model achieves higher results at each point of the PR curves. The regions with standard deviation do not overlap, which indicates a high statistical significance of the performance difference between the methods.
Positive predictive values (also PPV) with four selected thresholds, i.e., top-10, 20, 50, & 100 pHLA pairs selected by each method. For example, the score for PPV at top-10 describes what fraction of hits can be expected by testing in the laboratory 10 pHLA pairs with the highest rank from each method.
Identify the right extracellular protein targets
by modeling the HLA-II presentation pathway
Consider the presentation pathway
of HLA-II restricted peptides
in the development of cancer therapies
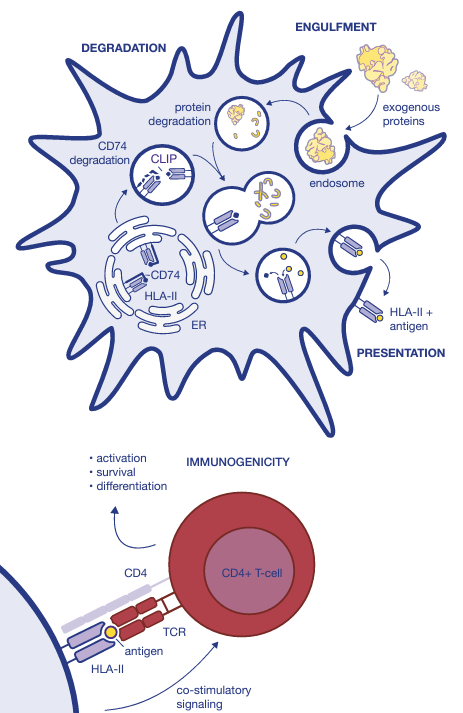
Important role
of CD4+ T-cells...
● Tumor eradication depends on the proper activation of both CD8+ and CD4+ T-cells.
● Anti-tumor CD4+ T-cells can directly eliminate tumor cells and orchestrate local immune responses, supporting the activity of other cells.
● When only CD8+ are activated, escaping the immune response by the tumor is more common than with both CD8+ and CD4+ activations. Moreover, some cancer types (for instance, CRC) tend to downregulate HLA-I expression while upregulating it for HLA-II.
● The structure of HLA-II allows for binding longer and more diversified peptides than HLA-I.
● The lack of immunogenic HLA-II antigens may cause unresponsiveness to immunotherapy in patients with immunogenic HLA-I antigens.
Ardigen's ARDisplay-II model enables prediction of HLA-II presented peptides with over 2 times higher Average Precision than standard solutions4
The study cohort5 includes patients with GBM (glioblastoma) and induced presentation.
The dataset is comprised of 17 distinct alpha & beta chains of corresponding HLA class II canonical alleles (loci DQ, DP, and DR). The population coverage3 shows that the study cohort is representative and shares at least three alpha/beta chains with almost 80% of the worldwide population.
Comparison of precision-recall (PR) curves. Our model achieves higher results at each point of the PR curves. The regions with standard deviation do not overlap, which indicates a high statistical significance of the performance difference between the methods.
Positive predictive values (also PPV) with four selected thresholds, i.e., top-10, 20, 50, & 100 pHLA pairs selected by each method. For example, the score for PPV at top-10 describes what fraction of hits can be expected by testing in the laboratory 10 pHLA pairs with the highest rank from each method.
Identify the right protein targets
with cross-reactivity check
Address off-target toxicity in cancer
immunotherapies long before it happens
There are different strategies for boosting the immune system to find and destroy harmful germs or cells, including tumor cells. Such immunosurveillance is possible due to T-lymphocytes’ recognition of epitopes presented via HLA molecules. If you know which peptides are on the cell surface, you can use them as targets in adoptive cell therapies (TILs or TCR-engineered T-cells).
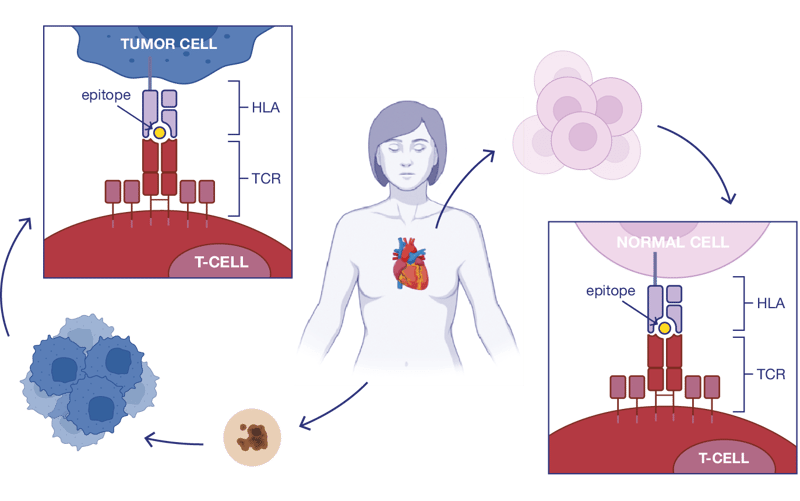
Typically, T-cells can naturally recognize more than one epitope as foreign. Therefore, off-target toxicity is a key issue to consider when developing immunotherapies. To select the safest peptide targets, support your research with computational immunology and Ardigen's ARDitox platform (patent pending,see EP22461636).
Did you know...?
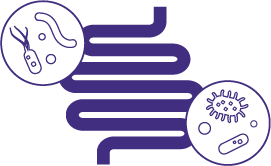
Cross-presentation of exogenous peptides can lead to the presentation of epitopes of the intestinal microbiota and other bacteria on the cell surface. This can result in the development of allergies and autoimmune diseases.
Monitoring the cross-reactivity in patients based on whole exome sequencing results combined with microbiome analysis may help to explain these health problems and enable the design of specific therapies.
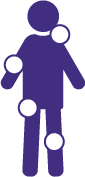
The development of autoimmune diseases can be related to molecular mimicry; a mechanism caused by T-cells interacting with both self-antigens and viral antigens.
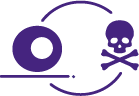
Off-target toxicity leading to side effects of variable intensity - from mild reactions to severe ones - is a common cause of clinical trial failure in cancer immunotherapies. The severity of these reactions strongly depends on the type of tissue affected. The most common side effects are skin reactions, flu-like symptoms, organ inflammation, and changes in mental status. There are clinical trials reported where it has led to coma or even death.
Ardigen's ARDitox platform is a powerful tool for augmenting toxicity evaluation designed to improve cancer immunotherapy development. This computational approach is ideal for screening target epitopes to assess the risk of potential off-target toxicity.
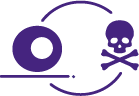
Off-target toxicity
Identify potential off-target toxicities in cancer immunotherapies
with Artificial intelligence to improve safety, and speed up therapy development

Check how we stand out
from other solutions
- ● We cover a larger space of peptides, as in other methods the number of permitted mismatches between the target peptide and the potential off-target epitopes is limited to only a few amino acids. This factor is the most important in safety evaluation.
- ● Extend the reference proteome (of the human population or your study cohort) with frequently occurring mutations to make sure you do not miss targets present in some human sub-population.
● Get a higher performance thanks to the ARDisplay model, our custom deep-learning presentation model trained
on mass-spectrometry data that outperforms standard models like MHCflurry or netMHCpan.
● Recognize TCR-facing amino acids (epitope) from HLA-facing ones (agretope). Incorporate this information while comparing the target peptide with putative off-targets and determine the risk of cross-reactivity based on the physico-chemical properties of the selected amino acids.
● User-friendly dashboard for the inspection of the generated mRNA and protein expression levels of the identified cross-reactive peptides
Exemplary results
The MAGE-A3 specific T-cells infused to patients during a clinical trial did recognize not only the targeted epitope, but also a Titin (TTN) peptide. The recognition of this peptide presented on healthy heart tissue has led to two fatalities.
With the ARDitox platform being tested retrospectively, we were able to anticipate the danger.
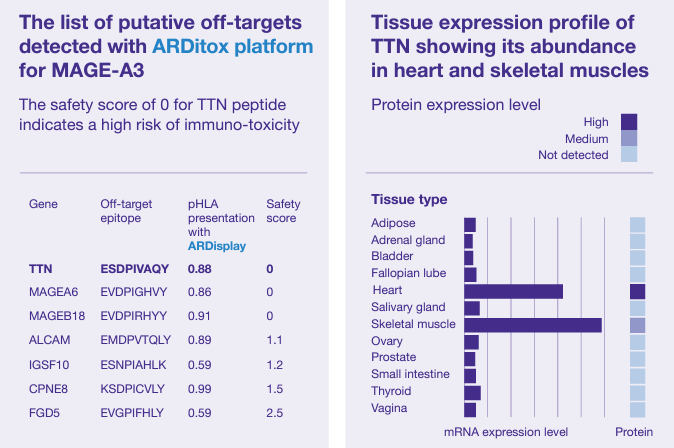
Identify the right protein targets
possible applications of our tools
Possible issues when using
openly available tools
● Openly/academically available tools are not adjustable to peptides from alternative sources. There is no easy way to check the model applicability nor adjust it to your domain.
● There are almost 25k alleles known for canonical HLAs (HLA-A, -B, and -C). Most of them are not well studied, and standard models are not adapted to them and lack problem-specific solutions.
● Predictions of binding affinity (BA) and presentation probability (EL) are highly correlated with Pearson correlation coefficient ρ=0.747.
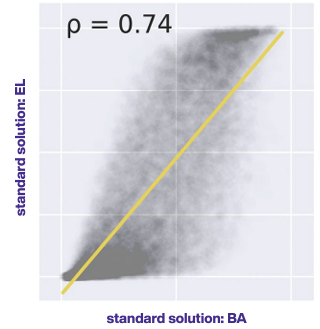
Our expertise & technology tailored to your needs
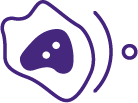
Explore targets from alternative
cancer germline transcripts
Indicate short peptides from
diverse minigene-encoded or
lentiviral-derived epitopes that
end up on the cell surface
Identify the patient's HLA alleles
presenting a given peptide
Predict whether your peptide can
be presented on multiple HLAs
Determine agretopic parts for
a peptide with a given HLA allele
Determine binding cores of
HLA-II restricted peptides
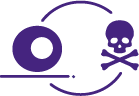
Predict potential off-target
toxicity long before it happens
Search for mimotopes to develop
vaccines against viral diseases -
find peptides mimicking your
target epitope by comparing their
structural & functional similarities
Identify mimotopes that can lead
to allergies caused by patient
epitome cross-reactivity
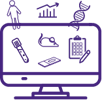
Integrate data from different
types of experiments so that
every relevant biological aspect
is addressed
Examine the influence of different
proteasome subunits and
peptidases on pools
of truncated peptides
Build in silico peptide library and
detect only promising targets to
validate experimentally, thus
overcoming the problem of
labor-intensive deconvolution
Overcome the limitations of
laboratory screening by easily
incorporating all scanning
approaches at once8
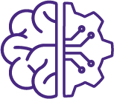
Use reliable predictions with
domain applicability check
and model confidence verification
Adjust & finetune our AI-based
solutions to your specific needs
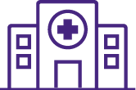
Identify unmet clinical needs
and make recommendations for
treatment approaches
1 Both models were trained on eluted ligands detected by MS experiments. The ARDisplay-I model differs a lot from standard solutions for EL prediction; for top-100 pHLA pairs, on average, there are only 14-18 samples shared (25th & 75th percentile, respectively).
2 Löffler et al., Mapping the Ligandome of Colorectal Cancer Reveals Imprint of Malignant cell transformation, Cancer Research 78.16 (2018): 4627-4641.
3 HLA class I & II population coverage based on http://tools.iedb.org/population/
4 Both models were trained on eluted ligands detected by MS experiments. The ARDisplay-II model differs a lot from standard solutions for EL prediction; for top-100 pHLA pairs, on average, there were 12士3 samples shared.
5 Forlani et al., CIITA-Transduced Glioblastoma Cells Uncover a Rich Repertoire of Clinically Relevant Tumor-Associated HLA-II Antigens, 2021.
6 Expitope 2.0, iVax (JanusMatrix), Dhanik, et al. (2016), or Lee et al. (2020).
7 Mazzocco et al., AI-aided design of epitope-based vaccine for the induction of cellular immune responses against SARS-CoV-2, 2021.
8 for instance, alanine scanning, random replacements, scrambled, truncated, positional, overlapping.
Are you interested in Ardigen's Immunology solutions?
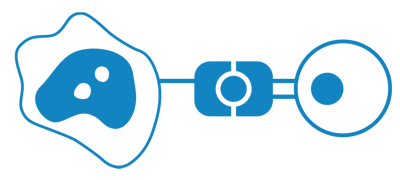
Immunity by design
Whether you are working on TCR-Ts, TCRm-Abs, peptide-based vaccines, or other immunotherapy approaches, we would be excited to learn more about your projects and explore how we can support you.