Case Studies, Publications
& Webinars
The science of today is the technology of tomorrow — Edward Teller
Scientific Posters
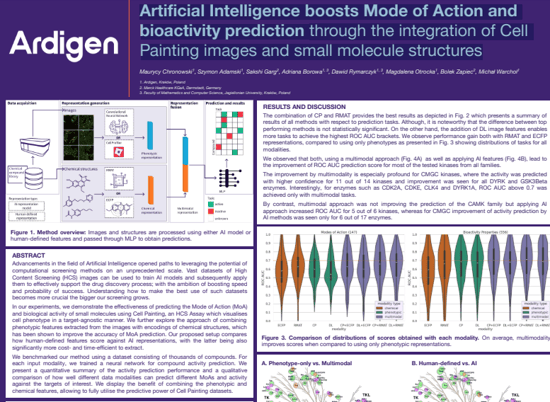
MoA & Bioactivity prediction
SLAS Europe 2024
Artificial Intelligence boosts Mode of Action and bioactivity prediction through the integration of Cell Painting images and small molecule structures
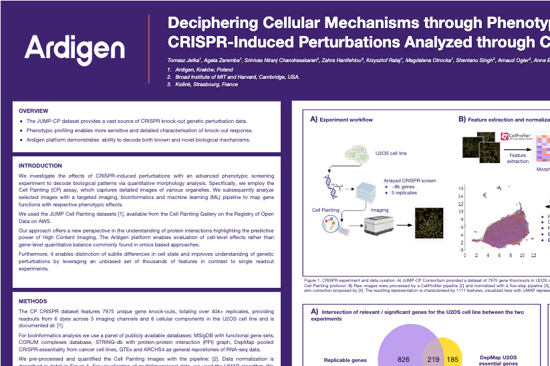
Deciphering Cellular Mechanisms through Phenotype
SLAS US 2024
CRISPR-Induced Perturbations Analyzed through Cell Painting and Machine Learning
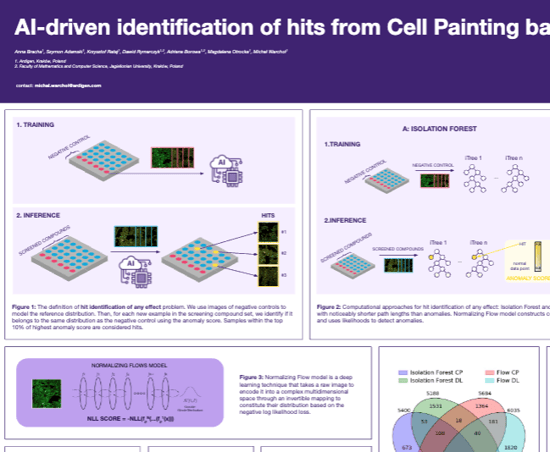
Identification of hits - any effect
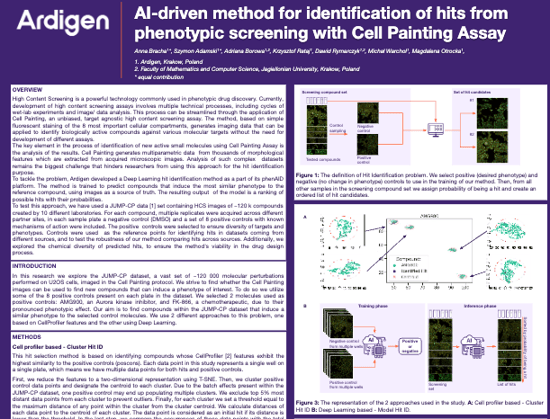
Identification of hits - target effect
SLAS Europe 2023
AI-driven method for identification of hits from phenotypic screening with Cell Painting Assay
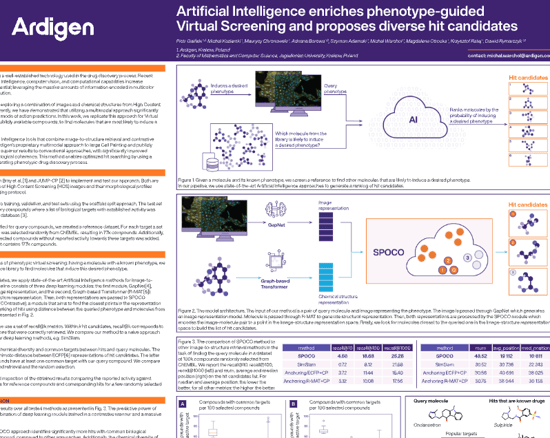
Phenotype-guided Virtual Screening
SLAS USA 2023
Artificial Intelligence enriches phenotype-guided Virtual Screening and proposes diverse hit candidates
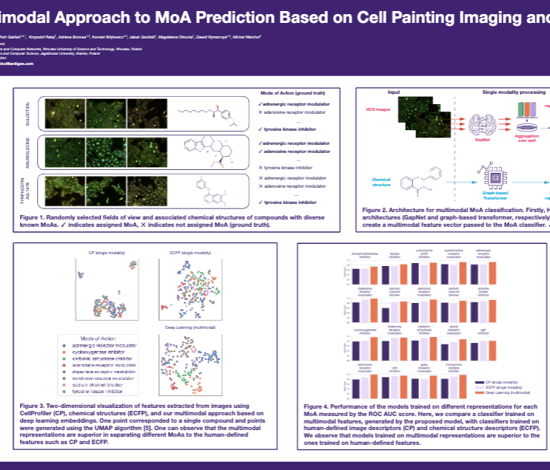
Multimodal Approach to MoA Prediction
ELRIG 2022
Multimodal Approach to MoA Prediction Based on Cell Painting Imaging and Chemical Structure Data
Webinars
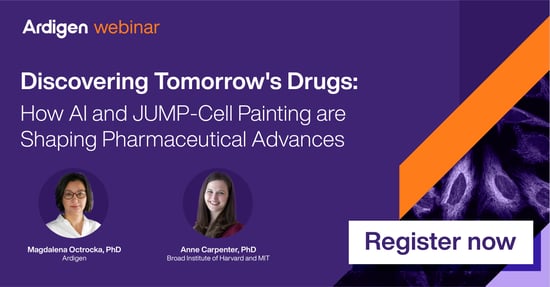
How AI and JUMP-Cell Painting are Shaping Pharmaceutical Advances
Explored of the JUMP-Cell Painting Consortium's impact on drug discovery, detailing its data-driven approach using cellular imaging and analytics to enhance the pharmaceutical pipeline, and featured insights from Dr. Anne Carpenter on the innovative PhenAID JUMP-CP Data Explorer.
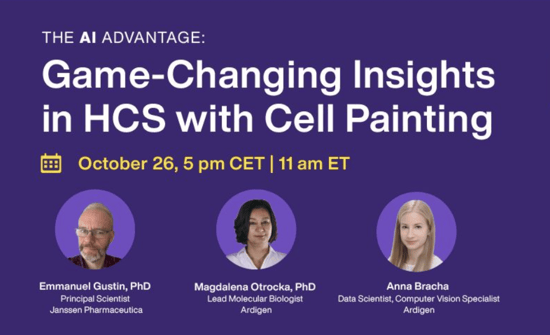
Game-Changing Insights in HCS with Cell Painting
Discover how AI and High Content Screening, especially with Cell Painting Assay, can revolutionize drug discovery by enhancing efficiency, accuracy, and insight.
Publications
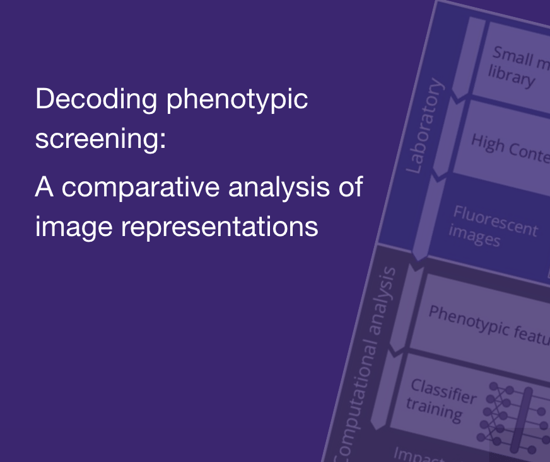
Decoding phenotypic screening: A comparative analysis of image representations
Biomedical imaging techniques like high content screening (HCS) are vital for drug discovery but are costly and mainly used by pharmaceutical companies. The JUMP-CP consortium addressed this by releasing a large open image dataset of chemical and genetic perturbations, aiding deep learning research. This study uses the JUMP-CP dataset to create a universal representation model for HCS data from U2OS cells and the CellPainting protocol through supervised and self-supervised learning.
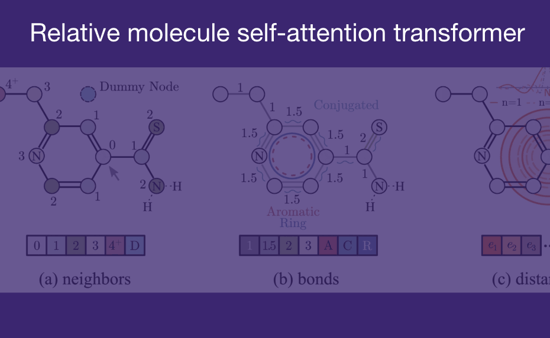
Relative molecule self-attention transformer
The Relative Molecule Self-Attention Transformer employs relative self-attention and 3D molecular representation to enhance molecular property prediction. Its two-step pretraining procedure optimizes hyperparameters effectively, achieving performance comparable to state-of-the-art models on various downstream tasks.